
- Developing a comprehensive AI strategy involves assessing AI readiness, identifying high-impact use cases, and aligning AI initiatives with business goals to maximize value creation.
- Key components include conducting an AI readiness assessment, building a robust data infrastructure, defining clear AI vision and objectives, and ensuring ethical and responsible AI use.
- Continuous learning, change management, and robust AI security measures are essential for successful AI implementation and long-term organizational transformation.
According to a recent survey, 66% of companies now consider AI critical to their success. In this guide, we'll walk through the essential steps to develop a comprehensive enterprise AI strategy for 2024. We'll cover everything from laying the groundwork to measuring success, ensuring you're well-equipped to lead your organization into the AI-driven future.
Foundations of enterprise AI strategy
Let's kick things off by laying the groundwork for your AI journey. Developing a solid enterprise AI strategy isn't just about jumping on the latest tech bandwagon. It's about creating a roadmap that aligns with your business goals and leverages your organization's unique strengths. We'll explore how to assess your AI readiness, identify high-impact use cases, and set the stage for successful implementation.
Conducting a comprehensive AI readiness assessment is essential to evaluate your current technological infrastructure and data assets. This assessment helps you understand your organization's strengths, weaknesses, and areas for improvement. By evaluating existing data infrastructure, including data quality, accessibility, and integration capabilities, you can identify gaps that need to be addressed. Additionally, assessing your current AI and machine learning capabilities within the organization will give you a clear picture of where you stand.
It's also important to analyze the skill sets of your workforce and identify gaps in AI-related expertise. This will help you understand the training and hiring needs required to support your AI initiatives. Reviewing your current IT systems and their compatibility with AI technologies is another crucial step in the readiness assessment.
Identifying and prioritizing AI use cases based on potential business value and strategic alignment is another critical aspect of laying the groundwork. Conducting workshops with business units to brainstorm potential AI applications can help you identify high-impact use cases. Developing a scoring system to evaluate use cases based on feasibility, impact, and strategic alignment will help you prioritize initiatives that offer significant business value. Creating proof-of-concept projects for top-ranked use cases to validate assumptions and establish a pipeline of AI initiatives can further ensure success.

A survey by Veritone found that "52% of companies accelerated their AI adoption plans due to the Covid crisis", highlighting the growing importance of AI in business strategies.
Defining AI vision and objectives
Now that we've laid the foundation, it's time to get specific about what you want to achieve with AI. Your AI vision should be a north star, guiding all your AI initiatives. We'll discuss how to craft a compelling AI vision and set measurable objectives that align with your overall business goals.
Articulating a clear and inspiring AI vision that aligns with the company's mission and values is crucial. This vision should resonate with stakeholders across the organization and provide a sense of direction. Setting SMART (Specific, Measurable, Achievable, Relevant, Time-bound) objectives for AI initiatives ensures that your goals are clear and attainable. Involving key stakeholders from various departments in defining AI objectives is essential to ensure buy-in and support.
Creating a roadmap that outlines short-term wins and long-term AI goals helps maintain momentum and demonstrates progress. This roadmap should include milestones and deliverables that align with your overall business strategy.
Conducting AI readiness assessment
Before diving headfirst into AI implementation, it's crucial to understand where you stand. An AI readiness assessment helps you identify your strengths, weaknesses, and areas for improvement. We'll explore the key components of this assessment and how to use the results to inform your strategy.
Evaluating existing data infrastructure, including data quality, accessibility, and integration capabilities, is a key component of the readiness assessment. This evaluation helps identify gaps and areas that need improvement. Assessing your current AI and machine learning capabilities within the organization provides insights into your strengths and weaknesses. Analyzing the skill sets of your workforce and identifying gaps in AI-related expertise helps determine the training and hiring needs required to support your AI initiatives.
Reviewing your current IT systems and their compatibility with AI technologies is another crucial step in the readiness assessment. This review helps identify any limitations or challenges that may need to be addressed before implementing AI initiatives.
Identifying high-impact AI use cases
Not all AI projects are created equal. To maximize your return on investment, you need to focus on use cases that offer significant business value. We'll discuss strategies for identifying and prioritizing AI initiatives that align with your strategic objectives and have the potential to drive real impact.
Conducting workshops with business units to brainstorm potential AI applications can help identify high-impact use cases. Developing a scoring system to evaluate use cases based on feasibility, impact, and strategic alignment ensures that you prioritize initiatives that offer significant business value. Creating proof-of-concept projects for top-ranked use cases to validate assumptions further ensures success. Establishing a pipeline of AI initiatives, balancing quick wins with long-term transformational projects, helps maintain momentum and demonstrate progress.
Building a data-centric foundation
Data is the lifeblood of AI. Without high-quality, accessible data, even the most sophisticated AI models will fall short. In this section, we'll delve into the critical aspects of building a robust data infrastructure to support your AI initiatives.
Developing a comprehensive data strategy that addresses data collection, storage, and management is essential. This strategy should include data governance policies to ensure data quality, security, and compliance. Implementing data integration tools to unify disparate data sources across the organization is crucial for creating a cohesive data ecosystem. Establishing data quality metrics and monitoring processes helps maintain data integrity and ensures that your AI models are based on accurate and reliable data.
Data strategy and governance
A solid data strategy is the backbone of successful AI implementation. We'll explore how to develop comprehensive data management practices that ensure data integrity, security, and compliance. This isn't just about following rules – it's about creating a data-driven culture that fuels your AI ambitions.
Creating a data governance committee with representatives from IT, legal, and business units helps ensure that data management practices align with organizational goals. Developing data quality standards and implementing automated data cleansing processes helps maintain data integrity. Establishing data access controls and audit trails ensures data security and regulatory compliance. Implementing metadata management tools improves data discoverability and understanding, making it easier to leverage data for AI initiatives.
Unifying data silos
Data silos can be a major roadblock to AI success. We'll discuss strategies for breaking down these barriers and creating a cohesive data ecosystem. By integrating disparate data sources, you'll unlock the full potential of your AI applications and drive more accurate insights.
Conducting a data inventory to identify all data sources across the organization is a crucial first step. Implementing a data lake or data warehouse solution to centralize data storage helps create a cohesive data ecosystem. Utilizing ETL (Extract, Transform, Load) tools to standardize data formats and structures ensures that data is consistent and reliable. Developing APIs to enable seamless data exchange between systems and applications further enhances data integration and interoperability.
Aligning AI with business strategy
AI shouldn't exist in a vacuum. To truly drive value, your AI initiatives must be tightly integrated with your overall business strategy. We'll explore how to ensure that every AI project contributes directly to your core business objectives and creates tangible value.
Mapping AI initiatives to specific business KPIs and strategic goals ensures that your AI projects align with organizational priorities. Developing a value realization framework to track and measure the impact of AI projects helps demonstrate the value of AI initiatives. Establishing cross-functional teams to bridge the gap between AI development and business operations ensures that AI projects are aligned with business needs. Implementing agile methodologies helps ensure that AI projects remain flexible and responsive to evolving business requirements.
A recent "EY's 2024 AI Pulse Survey reveals that among organizations investing in AI, these investments are delivering positive returns", especially in areas like operational efficiencies (77%), employee productivity (74%), and customer satisfaction (72%).
ROI-driven AI deployment
Let's talk money. AI projects can be resource-intensive, so it's crucial to prioritize initiatives based on their potential return on investment. We'll discuss how to evaluate the ROI of AI projects and make data-driven decisions about where to allocate your AI resources.
Developing a standardized ROI calculation methodology for AI projects helps ensure consistent and accurate evaluations. Implementing a portfolio management approach to balance high-risk, high-reward AI initiatives with more conservative projects ensures a balanced and sustainable AI strategy. Establishing key performance indicators (KPIs) to measure the success of AI deployments helps track progress and demonstrate value. Conducting regular reviews to assess the financial impact of ongoing AI projects and adjust strategies accordingly helps ensure that resources are allocated effectively.
AI technology stack and infrastructure
Now that we've covered the strategic aspects, let's dive into the nuts and bolts of AI implementation. Building the right technology stack and infrastructure is crucial for supporting your AI initiatives. We'll explore the key components you need to consider and how to design a scalable AI ecosystem.
Assessing cloud vs. on-premises infrastructure options for AI workloads is an important consideration. Each option has its advantages and trade-offs, so it's essential to evaluate which is the best fit for your organization. Evaluating different AI frameworks and libraries based on your specific use cases helps ensure that you choose the right tools for your needs. Considering the trade-offs between using pre-built AI solutions and developing custom models helps determine the best approach for your organization.
Selecting AI platforms and tools
With a plethora of AI platforms and tools available, choosing the right ones for your organization can be overwhelming. We'll break down the key factors to consider when evaluating AI technologies, including machine learning frameworks, cloud services, and development tools.
Conducting a thorough assessment of available AI platforms, considering factors like scalability, ease of use, and integration capabilities, helps ensure that you choose the right tools for your needs. Evaluating cloud-based AI services from major providers like AWS, Google Cloud, and Azure provides insights into the capabilities and limitations of each platform. Considering open-source AI frameworks such as TensorFlow and PyTorch for custom model development offers flexibility and customization options. Assessing the need for specialized AI hardware, such as GPUs or TPUs, for high-performance computing ensures that your infrastructure can support your AI workloads.
AI integration and interoperability
AI doesn't exist in isolation. For maximum impact, your AI systems need to play nice with your existing enterprise applications and workflows. We'll explore strategies for ensuring seamless integration and interoperability across your tech ecosystem.
Developing a comprehensive integration strategy that outlines how AI systems will connect with existing applications is crucial. Utilizing enterprise service buses (ESBs) or API gateways to facilitate communication between AI and legacy systems helps ensure seamless integration. Implementing data standardization practices to ensure consistent data formats across integrated systems enhances interoperability. Conducting thorough testing to verify the interoperability of AI solutions with existing workflows ensures that AI systems work seamlessly with your existing tech ecosystem.
AI governance and ethics
With great power comes great responsibility. As AI becomes more prevalent in your organization, it's crucial to establish frameworks and practices that ensure responsible and ethical use. We'll explore the key components of AI governance and how to navigate the ethical challenges of AI implementation.
Establishing an AI ethics committee to oversee the development and deployment of AI systems ensures that ethical considerations are at the forefront of your AI strategy. Developing clear guidelines for ethical AI use, addressing issues like bias, privacy, and transparency, helps ensure responsible AI deployment. Implementing regular AI audits to ensure compliance with ethical guidelines and regulatory requirements helps maintain accountability and trust.
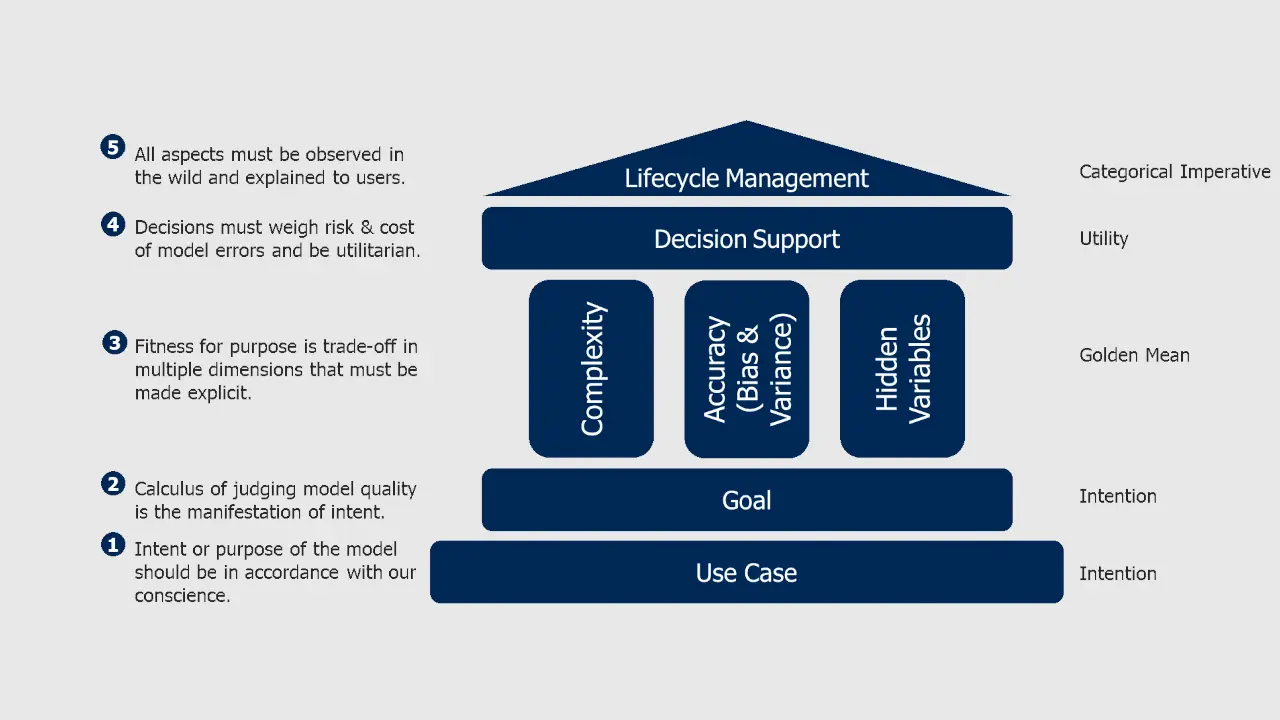
Ensuring AI transparency and explainability
Black box AI can be a tough sell, especially in regulated industries. We'll explore methods for making AI decision-making processes more transparent and interpretable, building trust with stakeholders and ensuring compliance with regulations.
Implementing model-agnostic explanation techniques like LIME (Local Interpretable Model-agnostic Explanations) or SHAP (SHapley Additive exPlanations) helps provide clear explanations for AI-driven decisions. Developing user-friendly interfaces that provide clear explanations of AI decisions to end-users enhances transparency and trust. Establishing a documentation process for AI models, including their training data, algorithms, and decision-making criteria, ensures that AI systems are transparent and accountable. Conducting regular audits of AI systems to ensure ongoing transparency and explainability helps maintain trust and compliance.
AI talent and organizational change
Your AI strategy is only as good as the people implementing it. In this section, we'll address the human element of AI strategy, including talent acquisition, skill development, and the cultural transformation needed to become an AI-driven organization.
Conducting a skills gap analysis to identify areas where AI expertise is lacking helps determine the training and hiring needs required to support your AI initiatives. Developing a comprehensive AI training program for existing employees helps build internal AI capabilities. Creating partnerships with universities and AI research institutions to foster talent pipelines helps ensure a steady supply of AI talent. Establishing clear AI career paths within the organization helps attract and retain top talent. Implementing a mentorship program pairing experienced AI professionals with junior team members helps build a strong AI team. Developing cross-functional training programs to ensure non-technical staff understand AI basics helps create a data-driven culture. Creating an internal AI knowledge base to facilitate continuous learning and best practice sharing helps maintain AI expertise and innovation.
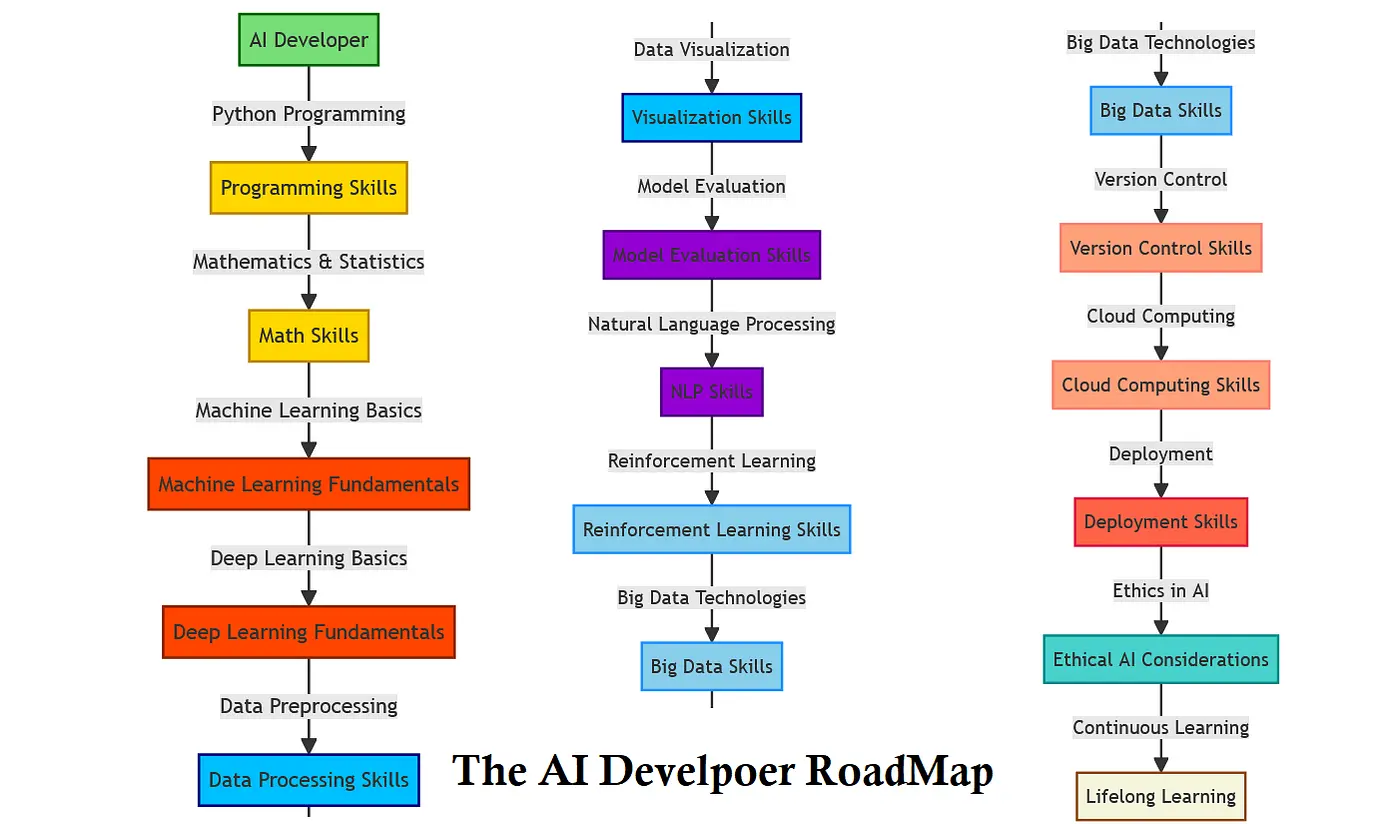
Building AI capabilities
Developing internal AI expertise is crucial for long-term success. We'll explore strategies for building your AI capabilities through hiring, training, and upskilling
Developing internal AI expertise is crucial for long-term success. We'll explore strategies for building your AI capabilities through hiring, training, and upskilling initiatives. Remember, it's not just about data scientists – you need a range of skills to support your AI ecosystem.
Establishing clear AI career paths within the organization can attract and retain top talent. Implementing a mentorship program that pairs experienced AI professionals with junior team members helps build a strong AI team. Developing cross-functional training programs ensures that non-technical staff understand AI basics and can collaborate effectively with AI teams. Creating an internal AI knowledge base facilitates continuous learning and best practice sharing, helping maintain AI expertise and innovation.
For more on developing effective prompts for AI systems, check out this Guide to Prompts. It can help teams enhance their ability to leverage AI tools effectively.
AI center of excellence
An AI Center of Excellence (CoE) can be a game-changer for your organization. We'll discuss how to establish a centralized team that drives AI innovation, promotes best practices, and facilitates knowledge sharing across your company.
Defining the structure and responsibilities of the AI CoE is the first step. Implementing a project intake process to evaluate and prioritize AI initiatives ensures that resources are allocated effectively. Developing standardized methodologies and tools for AI development and deployment helps maintain consistency and quality. Establishing key performance indicators (KPIs) to measure the impact of the CoE helps track progress and demonstrate value.
AI literacy programs
For AI to truly transform your organization, everyone needs to speak the language. We'll explore how to implement company-wide education initiatives that increase AI awareness and competency at all levels, from the C-suite to the front lines.
Developing role-specific AI training modules tailored to different departments and job functions ensures that everyone understands how AI impacts their work. Implementing gamification techniques makes AI learning engaging and interactive. Creating an AI resource library with curated content for self-paced learning provides ongoing education opportunities. Establishing an AI certification program recognizes and incentivizes AI proficiency, encouraging continuous learning.
Change management for AI adoption
Implementing AI isn't just a technical challenge – it's a people challenge. We'll discuss strategies to facilitate organizational acceptance and integration of AI technologies, addressing common fears and resistance to change.
Developing a comprehensive change management plan specifically for AI initiatives helps address concerns and build support. Implementing regular communication channels keeps stakeholders informed about AI progress and impact. Identifying and empowering AI champions across different departments helps drive adoption. Establishing feedback mechanisms to address concerns and iterate on AI implementation strategies ensures continuous improvement and acceptance.
AI champions network
Creating a network of AI advocates can significantly boost adoption across your organization. We'll explore how to identify and empower AI champions in different departments, fostering a culture of innovation and continuous learning.
Developing criteria for selecting AI champions based on technical aptitude and leadership skills ensures that the right individuals are chosen. Implementing a train-the-trainer program equips AI champions with the knowledge to educate others. Creating a community platform for AI champions to share experiences and best practices facilitates collaboration and learning. Establishing recognition programs incentivizes and rewards successful AI advocacy efforts.
Generative AI integration
Generative AI is revolutionizing industries, and it's time to consider how it fits into your strategy. We'll explore ways to incorporate generative AI technologies into your enterprise, unlocking new capabilities and opportunities for innovation.
Identifying potential use cases for generative AI across different business functions helps determine where these technologies can add value. Evaluating leading generative AI platforms and their integration capabilities with existing systems ensures seamless deployment. Developing guidelines for the responsible use of generative AI, addressing ethical concerns and potential risks, helps ensure responsible and ethical deployment.

Use cases for generative AI
Generative AI isn't just about creating art or writing content. We'll dive into practical applications of generative AI that can drive innovation and efficiency across various departments in your organization.
Exploring generative AI applications in product design and prototyping processes can accelerate R&D and innovation. Implementing generative AI for personalized marketing content creation at scale enhances customer engagement and reduces manual effort. Utilizing generative AI for automated code generation and software development assistance can streamline development processes. Investigating the use of generative AI in scenario planning and strategic decision-making helps organizations anticipate and adapt to future challenges.
Content creation and augmentation
Generative AI is transforming content creation. We'll explore how to leverage these technologies for automated content generation, translation, and personalization at scale, while maintaining your brand voice and quality standards.
Implementing fine-tuning techniques to adapt generative AI models to your specific brand voice and style ensures consistency in AI-generated content. Developing content workflows that combine AI-generated drafts with human editing and curation helps maintain quality. Utilizing generative AI for multilingual content creation and localization efforts expands your reach. Implementing content quality assurance processes ensures that AI-generated content meets standards and maintains your brand's integrity.
Product and service innovation
Generative AI can be a powerful tool for innovation. We'll discuss how to utilize these technologies to accelerate R&D processes, create new offerings, and stay ahead of the competition in your industry.
Implementing generative design techniques in product development processes can lead to innovative and optimized solutions. Utilizing generative AI for rapid prototyping and concept iteration accelerates the development cycle. Exploring the use of generative AI in creating personalized product recommendations and configurations enhances customer satisfaction. Investigating generative AI applications in predictive maintenance and service optimization can improve operational efficiency and reduce downtime.
Responsible generative AI deployment
With great power comes great responsibility. We'll discuss how to establish guidelines and safeguards for the ethical use of generative AI within your enterprise, addressing concerns about misinformation, copyright, and bias.
Developing clear policies for the use and attribution of AI-generated content ensures ethical and responsible deployment. Implementing content filtering mechanisms prevents the generation of inappropriate or harmful content. Establishing processes for human oversight and intervention in generative AI outputs ensures accountability. Conducting regular audits of generative AI systems ensures compliance with ethical guidelines and maintains trust.
Output verification and quality control
Ensuring the accuracy and appropriateness of AI-generated content is crucial. We'll explore processes and tools for verifying and quality-controlling generative AI outputs, maintaining trust and reliability in your AI-driven initiatives.
Implementing fact-checking algorithms to verify the accuracy of AI-generated information helps maintain credibility. Developing automated content moderation systems to flag potentially inappropriate or biased outputs ensures content quality. Establishing human-in-the-loop processes for final review and approval of AI-generated content ensures accountability. Implementing version control and audit trails for AI-generated outputs ensures transparency and traceability.
AI KPIs and metrics
You can't improve what you don't measure. We'll explore how to define key performance indicators that accurately measure the success and ROI of your AI initiatives, aligning them with your overall business objectives.
Developing a balanced scorecard approach for AI performance measurement, including technical and business metrics, ensures a comprehensive evaluation of AI initiatives. Implementing A/B testing frameworks to compare AI model performance against baseline methods helps identify improvements. Utilizing advanced analytics techniques to measure the indirect impact of AI on business outcomes provides a holistic view of AI performance. Establishing processes for regular reporting and visualization of AI performance metrics to stakeholders ensures transparency and accountability.
Model drift detection
AI models can degrade over time as the real-world data changes. We'll discuss techniques for identifying and addressing performance degradation in AI models, ensuring your systems remain accurate and reliable.
Implementing statistical methods for detecting data drift and concept drift in AI models helps identify performance degradation. Developing automated alerting systems to notify teams when model performance falls below thresholds ensures timely interventions. Utilizing ensemble methods to improve model robustness and detect anomalies in predictions enhances model reliability. Implementing versioning and rollback mechanisms for AI models ensures quick resolution of performance issues.
Continuous learning and improvement
The AI landscape is constantly evolving, and your strategy should too. We'll explore processes for ongoing refinement and evolution of AI systems based on new data, feedback, and technological advancements.
Implementing online learning techniques for real-time model updates based on new data ensures that AI systems remain current. Developing feedback loops to incorporate user interactions and corrections into model improvements enhances AI performance. Establishing a process for regular review of AI research and industry advancements informs strategy updates. Implementing transfer learning techniques to leverage knowledge from existing models for new use cases accelerates AI development and innovation.
A/B testing for AI
A/B testing isn't just for marketing – it's a powerful tool for optimizing AI performance. We'll discuss how to utilize experimental design techniques to fine-tune your AI models and enhance user experience.
Developing a framework for designing and executing A/B tests specifically for AI models ensures rigorous evaluation. Implementing multi-armed bandit algorithms for efficient exploration of model variations enhances optimization. Utilizing causal inference techniques to understand the true impact of AI model changes provides deeper insights. Developing processes for rapid iteration and deployment of successful AI model variations accelerates improvement.
AI security and risk management
As AI becomes more integral to your operations, it also becomes a potential target for bad actors. We'll address the unique security challenges and risks associated with enterprise AI deployment, ensuring your AI initiatives remain secure and resilient.
Conducting comprehensive risk assessments specific to AI systems and data helps identify vulnerabilities and threats. Implementing advanced encryption techniques for protecting AI models and sensitive training data ensures data security. Developing incident response plans tailored to AI-specific security threats ensures preparedness and quick resolution of security incidents.

AI-Specific cybersecurity measures
AI systems require a unique approach to security. We'll explore cybersecurity protocols tailored to protect AI systems and the data they process, addressing vulnerabilities specific to machine learning models and AI infrastructure.
Implementing model extraction defenses to prevent unauthorized access to AI model architectures ensures system integrity. Utilizing federated learning techniques to enhance data privacy in distributed AI systems protects sensitive information. Developing secure enclaves for sensitive AI computations and data processing ensures data security. Implementing robust authentication and access control mechanisms for AI systems and APIs ensures only authorized access.
Adversarial attack prevention
Adversarial attacks can compromise the integrity of your AI systems. We'll discuss strategies for developing defenses against attempts to manipulate or compromise AI models, ensuring the reliability of your AI-driven decisions.
Implementing adversarial training techniques to improve model robustness against malicious inputs enhances security. Developing input validation and sanitization processes specific to AI model inputs prevents attacks. Utilizing ensemble methods and model averaging to reduce vulnerability to single-point attacks enhances model reliability. Implementing runtime monitoring systems to detect and mitigate adversarial attacks in real-time ensures ongoing security.
AI risk assessment and mitigation
With AI comes new forms of risk. We'll explore how to identify potential risks associated with AI deployment and establish comprehensive mitigation strategies, ensuring your AI initiatives don't become liabilities.
Developing an AI-specific risk assessment framework considering technical, ethical, and business risks ensures comprehensive evaluation. Implementing scenario planning techniques to anticipate potential AI failures and their impacts ensures preparedness. Establishing clear lines of responsibility and accountability for AI-related risks within the organization ensures effective risk management. Developing contingency plans for AI system failures or unexpected behaviors ensures quick resolution and minimal disruption.
AI insurance and liability
As AI takes on more critical roles in your organization, it's important to consider the legal and financial implications. We'll explore insurance options and legal considerations related to AI implementation and potential failures.
Evaluating existing insurance policies for coverage of AI-related risks and liabilities ensures adequate protection. Exploring specialized AI insurance products that address unique risks of AI deployment provides additional security. Developing clear contractual agreements with AI vendors and partners regarding liability and indemnification ensures clarity and accountability. Implementing robust documentation practices to support potential legal or insurance claims related to AI systems ensures preparedness.
According to Deloitte's State of AI in the Enterprise report, "66% of respondents said that AI is critical to success, while 38% believe that using AI helps them differentiate from their competitors", highlighting the growing importance of a well-crafted AI strategy.
Leveraging Glean AI for your enterprise strategy
As we wrap up our comprehensive guide to creating an enterprise AI strategy, it's worth considering how specialized tools like Glean AI can support your initiatives. Glean AI offers a powerful platform that addresses many of the challenges we've discussed, from data integration to responsible AI practices.
Exploring Glean AI's advanced personalization features can enhance user experiences across your organization. Utilizing Glean AI's retrieval augmented generation capabilities improves the accuracy and relevance of AI-generated outputs. Leveraging Glean AI's turnkey implementation with over 100 connectors streamlines integration with your existing systems. Implementing Glean AI's responsible AI practices ensures ethical and transparent use of AI across your enterprise.
Ready to take your enterprise AI strategy to the next level? Discover how Glean AI can accelerate your AI initiatives while maintaining security, scalability, and flexibility. Contact us today for a personalized demo and see how Glean AI can transform your organization's approach to AI.
Key learnings and next steps
We've covered a lot of ground in this guide to creating an enterprise AI strategy for 2024. Let's recap the key learnings and outline some practical next steps to help you kickstart your AI journey.
Prioritizing alignment between AI initiatives and core business objectives maximizes value creation. Implementing robust data governance and infrastructure supports scalable AI deployment. Developing a comprehensive AI ethics framework ensures responsible and transparent AI use. Investing in continuous AI education and change management fosters organization-wide adoption.
For more on effectively utilizing AI-powered search capabilities, check out this How to search in Glean. It can help teams find and leverage information across the organization.
- Conduct a thorough AI readiness assessment to identify your organization's strengths and areas for improvement.
- Develop a clear AI vision and set of objectives that align with your overall business strategy.
- Establish a data-centric foundation by implementing strong data governance and integration practices.
- Build a scalable AI technology stack that can grow with your organization's needs.
- Create an AI governance framework to ensure ethical and responsible AI use.
- Invest in AI talent development and foster a culture of continuous learning.
- Implement robust AI security measures and risk management strategies.
- Continuously monitor and optimize AI performance using defined KPIs and metrics.
Remember, creating an effective enterprise AI strategy is an ongoing process. It requires commitment, flexibility, and a willingness to learn and adapt as the AI landscape evolves. By following the guidelines and best practices outlined in this guide, you'll be well-equipped to lead your organization into the AI-driven future.
As you embark on your AI journey, don't hesitate to seek expert guidance and leverage specialized tools like Glean AI to accelerate your progress. With the right strategy and resources, you can unlock the transformative power of AI and drive significant value for your organization. Schedule a demo today!