
- R
Generative AI and large language models (LLMs) are emerging as one of the most beneficial and transformative workplace technologies of our time. Capable of supercharging knowledge discovery and productivity, they’ll only become more essential as we try to keep up with the pace of competition.
If you’ve experimented with large language models before, however, you’ll know that they’re not perfect. While many of the results they provide are impressive in nature, they sometimes assertively present incorrect information as fact – as hallucinations. As more and more users attempt to integrate LLMs and generative AI into the workplace, it’s an issue that grows only more problematic at scale.
Many solutions, especially more generalized ones, struggle with a fundamental requirement for success – knowledge retrieval. Models don’t automatically understand which pieces of enterprise knowledge are most relevant for each user and query. Fine-tuning is also imperfect, often prohibitively expensive and resulting in inevitable errors when it comes to factual recall.
Tackling inaccuracy and inconsistency with RAG
This is where retrieval augmented generation (RAG) as a framework for generative AI comes in – by separating knowledge retrieval from the generation process via an external discovery system like enterprise search, LLMs and the responses they provide can be grounded on real, external enterprise knowledge that can be readily surfaced, traced, and referenced. This enables models to always have the right information at hand, while providing users with the confidence that the answers they’ve received aren’t merely hallucinations.
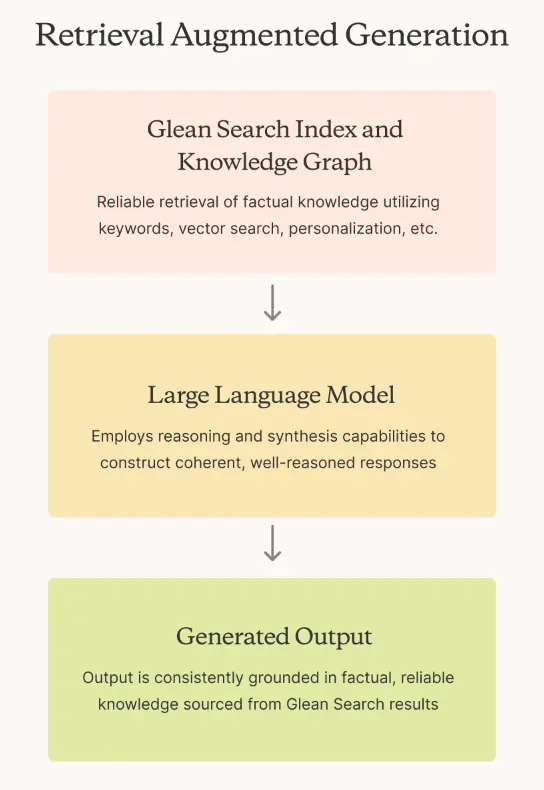
Once a query is received, the RAG process begins with the retrieval phase, where a knowledge retrieval solution searches for and surfaces information most relevant to the question at hand. It’s where knowledge management and retrieval engines shine – when it comes to internal knowledge, factors like relevancy, recency, and permissions also make RAG an ideal solution, since a good knowledge retrieval engine should be capable of already indexing information comprehensively with those elements in mind.
Once the proper information is surfaced, the model can now generate a response – which it excels in. Now armed with knowledge that’s relevant, recent, and referenceable, models can produce well-informed responses to queries grounded in real enterprise information, every time.
A best-in-class solution
Apart from RAG simply delivering more robust, trustworthy results, it’s also just not realistic to continuously retrain a model each time a new piece of information is added to a database. Fine-tuning may initially seem like an ideal solution, but it’s simply incapable of providing the deep understanding of enterprise information models needed to provide the best-possible answers in complex and fast-moving work environments.
For example, fine-tuning a model to answer nuanced questions respective to a specific workplace will require thousands of examples in order for it to provide a satisfactory resolution – even then, there’s still no guarantee that it won’t provide an entirely hallucinated answer sourced from generic training data. Without referenceability either, users will have no way to double-check and distinguish right from wrong.
RAG is the industry-leading solution for working with LLMs in an enterprise environment. Utilizing a robust knowledge retrieval system in tandem with an LLM is the most effective and cost-efficient way to ensure results are always relevant, recent, and permissions aware. Looking to learn even more about what RAG can do? Check out our additional resource on RAG here!
If you’re looking to get started with an enterprise-ready generative AI solution equipped with best-in-class workplace search and knowledge retrieval capabilities to fuel retrieval augmented general for the enterprise, sign up for a Glean demo today!